Abstract: As vast amounts of high-throughput data are generated from sequencing the microbiome, suitable analysis approaches are needed to effectively evaluate the data and reach robust conclusions to power studies incorporating microbiome research questions. Sequencing data are often represented as parts of the total sequencing effort, and therefore retain relative information to the other parts of the whole sequenced population. For microbiome data, sequenced reads are often assigned to annotations or are binned by sequence similarity, and the measurement of one taxon or gene is influenced by the other components measured. Due to this compositional nature of the data, common statistical analyses can produce misleading interpretations, and so compositionally- aware methodologies have emerged. We have adapted a compositional analysis framework into the evaluation and support of data generated in microbiome studies, and show applications of these approaches for evaluating product performance, and deriving insights from diverse sample types. We show these approaches, along with measures of biological variability and effect, can generate reliable conclusions from challenging conditions such as low biomass, rare taxa, or highly variable and sparse data.
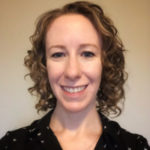
Jean M Macklaim
Bioinformatics Scientist