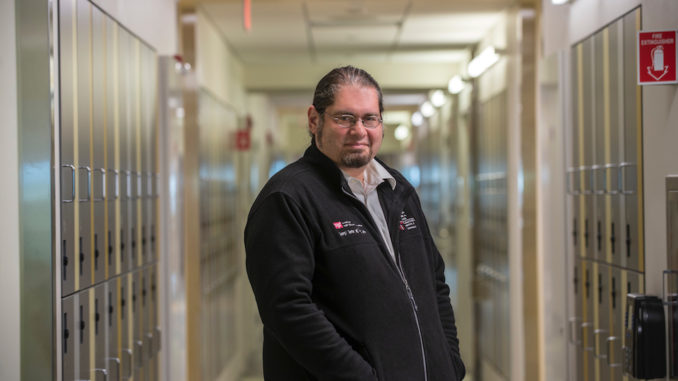
Many different chronic diseases have been associated with distinct gut microbiome ‘signatures’, but what has proved challenging to date is the translation of the compositional and functional microbiome differences into novel diagnostics and therapeutics. Moving into a new decade of microbiome research, the essential task—being undertaken by scientists around the world—is to give meaning to microbiome data and to connect it with relevant clinical parameters and outcomes.
Although the biostatistical and bioinformatic tools applied to microbiome data rarely garner the spotlight, they have enabled every microbiome study to date, no matter how small or big. Computational tools are indeed a crucial part of making meaning from microbiome data – and going forward, they will help identify the patterns in vast quantities of data that have potential relevance to clinical care.
Dr. Georg Gerber, MD, PhD,Assistant Professor of Pathology at Harvard Medical School, Chief of the Division of Computational Pathology at Brigham and Women’s Hospital and co-director of the Massachusetts Host-Microbiome Center, is one of the leading-edge microbiome computational biologists whose focus is on developing tools that will ultimately enable new microbiome-based diagnostics or therapeutics. Gerber’s lab colleagues span the range of expertise from computer science to physics to control theory, and they are working together to create novel computational methods, particularly in the machine learning area.
Microbiome Times editors caught up with Dr. Gerber to learn about his approach to microbiome data and how he works to develop new computational tools.
What is your general frameworkfor approaching microbiome data?
My lab focuses a lot on microbial dynamics. This is really key in a lot of the work we do.
For microbial dynamics, there are two complementary questions we ask.
The first is, can we predict what’s going to happen to the microbiome over time. For example if the microbiome is hit with antibiotics or a dietary change, can we predict what’s going to happen to the microbiome the next day or a week later?
Or we can turn that problem on its head, and ask: if we measure how the microbiome changes over time, can we predict what’s going to happen to the host? For example, if some microbes increased in your gut last week can we predict that you’ll get sick this week?
So at the broadest level, many of the models we develop try to answer these questions – predicting the microbiome or predicting from the microbiome.
Are the bioinformatics tools you develop unique to every situation?
There are recurrent themes for sure and we do try to develop tools that are fairly general purpose. On other hand, we always approach things from the perspective of answering specific scientific questions.
Coming up with a model that’s reasonably specific is going to be useful, in part because it’ll help you to efficiently answer the question you want to answer, but also because it can be easier to solve a specific problem.
That being said, we don’t want to do a one-off, solving one problem that’s very specific and very niche. So it’s a balance.
The human gut microbiome has been linked to so many different diseases. How do you decide which diseases or conditions are worth pursuing?
We think a lot about where to put resources because the microbiome is now such a diverse research and commercial space.
One of the filters we always start with when looking at indications is, first of all, how clear is the evidence for the role of the microbiome?
There are many areas where we have these associations and the microbiome could be a driver or it could not be, who knows.
So evaluating evidence for the association is important, certainly using animal models. I’m a big believer that they’re helpful in establishing the mechanism or at least a possible mechanism where the microbiome is driving the outcome. Whether it’s that exact mechanism in humans or not, won’t always be clear—but the concept that if a phenomenon in animals is caused by the microbiome, I am much more confident it could be a cause in people.
For instance, often times we’ll ask investigators—if they have an animal model and they think there might be a microbiome association—does antibiotic treatment change phenotype? If that’s the case,that starts to provide evidence. An even stronger avenue of evidence is whether there’s a plausible molecular pathway that could be involved either on the microbes’ side or on the host’s side.
For instance, in the case of food allergy, we have evidence in the animal model in terms of antibiotics affecting the course of food allergy, so that gives evidence the microbiome is important.We also have an understanding of the immunological mechanism on the host side. That’s a great starting point. The next step is to figure out how the microbiome interacts with that host mechanism.
The other thing as far as indications is the tractability of the disease itself. Diseases like IBD are so clinically complicated—to even define the disease, the heterogeneity is daunting. I do believe microbiome plays a role, but it’s very challenging to get down to mechanisms. There are so many other factors there.
Whereas if you’re looking at, say, an infection—colonization resistance—it’s pretty clear that the microbiome may have a direct impact. I also think some of the simpler immunological autoimmune conditions probably are going to be a lot more tractable in terms of actually developing a therapeutic.
Can you walk us through the process by which you might find a candidate microbiome therapeutic?
The first step is obviously figuring out the disease and indication. As I said, it’s really helpful to have ananimal model.
We work on a food allergy, which I think is a good use case here.
We have a human cohort, and we also have an animal model.
We have a pediatric cohort at risk for food allergy… looking over time is a key approach we take in all of our studies because this starts to allow us to establish causality rather than just association. In that cohort, looking over time, we asked: what changes in the microbiome are associated with the change in phenotype — meaning a kid becoming food allergic or staying non-food allergic.
We applied computational models to data from this cohort and that gave us a candidate list of potential microbes that could be protective or make food allergy worse.
So then we went in and developed a set of microbes from reference strain collections that were closest to those on our list and tested these in our animal model. We were then able to narrow it down to a defined group of bugs that prevents or treats food allergies. So then we had a potential therapeutic. From there, we can ask questions like: what’s the minimal set of bugs that’s still protective? We can use our computational models to help answer that and also what’ll happen if we introduce these bugs to an existing microbiome? How will the therapeutic microbes interact with the bugs already there?
With the food allergy project we got to a candidate bacterial therapy, which we’ve shown works in animal models, and now it’sbeen licensed to a company that ultimately wants to do a clinical trial with it.
For therapeutics, where does the mechanism of action come in?
Figuring out which specific bugs have the therapeutic effect is the first step, but then we want to drill down further to try and figure out what the underlying molecular mechanisms are.
We’re working on developing a combined experimental and computational platform so we can really do this at scale. Going from a particular host phenotype of interest, and looking at a large collection of microbes and perhaps theirmetabolites or other components, and computationally linking these to the host phenotypes.
As far as developing products, I think in some cases the bacterial therapy is the best way to go.In other cases it might actually be a small molecule.
From a scientific perspective we want to know what that mechanism is, but even just practically for thinking about therapy. There are obviously advantages to making and delivering small molecules if you can do it that way.
For diagnostics, is mechanism equally important?
We have a project going on like that in C. diff infection, trying to come up with, at the end of the day, a diagnostic for who’s going to recur or not.
From my perspective it would be ideal if the diagnostic had a mechanistic basis. From our animal model, we think that’ll be possible. But it could be that the most effective biomarker isn’t so clearly mechanistically linked. It might be that something upstream or downstream of the direct mechanism is actually the most robust signal.
You’ve been a pioneer in the new field of ‘computational pathology’. In this field, how are you working to advance medical research?
Computational pathology as a discipline is very broad. Pathology itself is really the foundation of modern scientific medicine, the idea that we’re trying to understand the mechanism behind disease and that’s going to lead to the best diagnosis and treatment.
Within my institution, Brigham and Women’s Hospital, I’m leading a new division where the vision is to leverage advanced computational tools—not off-the-shelf tools, but focusing where there’s really hard computational problems and innovation is needed—to understand disease mechanism so we can develop better diagnostics and therapeutics.
Specifically what we’re focusing onare three areas.
The first is imaging:using computational methods to analyze microscopy images to improve diagnosis and prognosis.
The second is computational immunology. This can drive a lot of innovations that are just now becoming feasible – partiallyenabled by next-gen and by other high-throughput modalities –with linking what’s happening in the immune system to host phenotype, such as how the immune response changes outcomes in cancer.
The third area is microbiome. The hope is we’ll actually have synergy between these different areas, because obviously, immunology and microbiome are intimately linked. But there’s also an important link with imaging, using new technologies where we can start addressing, for instance, interactions in space within tissues. We can look in situ. What genes is the host expressing in different parts of the tissue? How are host immune cells talking to other host cells? And how are microbes involved in all this in the spatial context? Using all this information and integrating it computationally we think we can get better insights into disease mechanisms and from there ultimately create better diagnostics and therapeutics. So that’s the ‘grand vision’ of what we’re trying to do in the new computational pathology division.
This interview has been edited for clarity and length.
