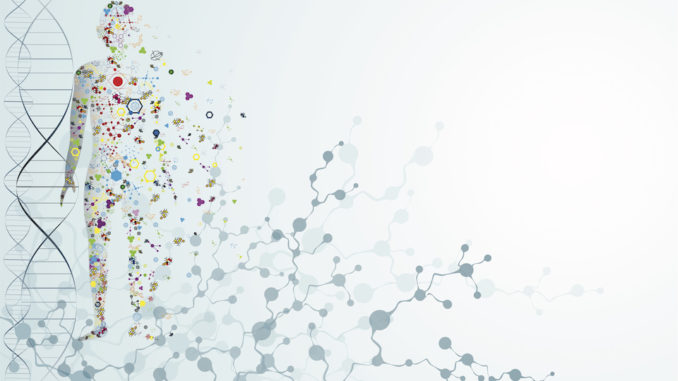
Hundreds of companies globally, funded by over USD $5.9 billion in venture capital to date, are now focused on leveraging the close relationship between humans and their resident microbes. And with new scientific publications on a weekly basis confirming links between the human microbiome and important aspects of health, in particular metabolism and immunity, human-associated communities of microbes provide a lush landscape for discovering novel drug substances and nutritional ingredients with potentially novel mechanisms of action.
At this stage, researchers in academia and industry have amassed a great deal of microbiome data but often struggle to identify promising therapeutics. Each microbiome of the human body – gut, skin, oral, or other – is an enormously complex, interconnected community of billions of microorganisms constantly blooming and dying, experiencing pressures from the human host which may change from moment to moment. But this complicated mix may contain the key microbial actors that make a difference to human health. So how is it possible to identify them?
“Therapeutic discovery proceeds in many different ways when it comes to the microbiome,”
says Prof. Gene Tyson, Director of the Centre for Microbiome Research at QUT and co-founder of the microbiome analysis and discovery company Microba Life Sciences.
“What’s exciting is that we find ourselves at a time when we can use advanced technologies in a systematic way to find signals in microbiome datasets.”
The signals or patterns discoverable in microbiome data may be bacterial taxa, microbial functional pathways, or even nutritional signals specifically related to gut microbes—and they are a first step for developing novel products to improve human health.
Tyson, along with Microba CSO A/Prof. Lutz Krause offer guidance on how to find more accurate signals and stronger leads in microbiome datasets for developing novel interventions.
Start with human data.
Tyson has observed that research scientists studying the microbiome can all too easily be misled by candidate therapeutic microorganisms that grow easily outside the human body and show strong effects in animal models of disease.
“Rather than focus on microbial targets with therapeutic value in animal models that might turn out to be rare in the human microbiome, or that act through different mechanisms in people, it’s preferable to start with microbes that appear to be meaningful in human disease,”
he says. Thus, human cohorts are required as a starting point.
Look for signals in large databases of human microbiome samples.
“With a large number of human metagenomes, it becomes possible to pull out signals from the microbiome and then to take them into the clinic to assess whether they are meaningful,” explains Tyson.
As it turns out, Microba has spent the past three years amassing one of the world’s largest gut microbiome datasets, called the Microba Discovery Database. Krause says,
“Our dataset currently contains more than 8,000 gut metagenomes paired with extensive metadata—diet, medications, medical history, and other factors.”
The company has collected up to 2,000 metadata points per sample, says Krause, with the size of the database allowing researchers to correct for common confounders such as gender, body mass index, and antibiotic usage.
He adds,
“From this database, we have found signals from the microbiome that others are missing. Using our leading discovery platform, we’ve found the same signals in publicly available datasets that we see in the larger Microba dataset, which have been missed by the people who generated the original data.”
Invest in high-resolution microbiome measurement.
Both Tyson and Krause maintain that researchers looking for potential therapeutics must capture the full complexity of the microbiome, including previously uncharacterized microbes, to identify the species, genes, and pathways that are critical to their research questions.
“The high resolution that shotgun metagenomics provides is critical,” says Tyson. “Very closely related species can have very different functions. We have seen that one species can have a strong therapeutic effect whereas a close relative does not.”
According to Krause,
“Where the real ‘magic’ happens at Microba is in the ability to accurately assign the metagenome reads to our robust genomic database. We have this amazing reference database which considers over 400,000 genomes, and will soon be expanding to consider over 1 million genomes. Once we generate the compositional analysis of the microbiome, we can make inferences from that genomic information around the important organisms in various health and disease states.”
The unique feature of the company’s comprehensive genome reference database is the number of uncultured species it contains. While other reference databases may fail to capture the approximately 70% of gut microbial species that have not been cultured so far, even those that are highly prevalent, Microba’s database with its high number of metagenome-assembled genomes (or MAGs) allows interrogation of the novel gut microbiome—allowing researchers to see microbial signals that may have been overlooked in other, narrower, analyses.
Ensure your microbiome measurements match reality.
“If you’re not measuring the gut microbiome correctly, then you’re going to miss a lot of signals,” says Tyson. This means using a microbiome analysis pipeline that matches ‘ground truth’, as confirmed by mock communities that are produced internally or by third parties.
Krause confirms,
“We have a highly evaluated pipeline. Our team has put a lot of effort into evaluating all the different tools and steps to ensure we get accurate results. We can correctly identify the signals that come out of mock communities.”
The sample processing incorporates a high level of automation and operates to ISO 15189 standards, with best-practice sequencing run quality control metrics.
Advanced artificial intelligence (AI) and machine learning.
The latest AI and machine learning techniques are indispensable for identifying the signals from a microbiome dataset, since associations between disease and microbes tend to be complex, sparse, and multivariate.
As a recent example, Microba took data from a group of 638 individuals with and without anxiety, and used multivariate statistics to find microbial species signatures associated with anxiety. Their analysis of this cohort helped the team identify promising microbial candidates important for anxiety, laying the groundwork for future therapeutics.
Further, the team used advanced machine learning methods to investigate whether gut microbiota data could discriminate between people with and without anxiety. To do this, Microba’s data scientists split the patient cohort into a training set and a test set, then optimized a range of models on the training set. From there, the optimal model was selected and evaluated on the test set. This analysis showed higher order non-linear relationships between the gut microbiota and anxiety, and the model predicted anxiety status with high accuracy (AUC = 0.88).
Mapping out the next steps in development
Once a microbiome signal is identified, however, many more steps must be taken to advance a potential live biotherapeutic product (in other words, a live-microbe-based drug). The usual next step is to culture the organisms and begin testing them across a range of in vitro and animal assays that are relevant to the disease of interest.
With the leads identified via Microba’s precision analysis platform, in many cases the top therapeutic candidates are uncultivated species.
But Krause says this doesn’t have to be a stumbling block—because the company has developed methods to isolate previously uncultured microbial candidates through genomic functional analysis.
“We’re able to culture novel bacteria using our genome-guided isolation approach,” says Krause. “Using this technique, we predict from the genomes of our target species the optimal growth conditions, and we can enrich for them in a complex donor sample.”
Once the bugs reliably grow under the specified conditions, the researchers can look toward manufacturing and scale-up.
Beyond therapeutics
Krause emphasizes that the company’s platform has already been used not just for identifying candidate microbiome therapeutics, but also for identifying diagnostics of potential clinical relevance.
“We’ve taken the time to create therapeutic and diagnostic capabilities with broad utility,” says Krause. “No matter which condition is of interest to a client, they are equipped to get the insights they need out of their dataset.”
Tyson says,
“We are driven to get the clearest picture of what’s going on in the microbiome—this is the basis of the medical innovations that will undoubtedly come from microbiome analysis in the years ahead.”
Find out more about the latest approaches for microbiome analysis and product development in this webinar: Driving New Discovery with Precision Microbiome Analysis & Advanced AI.
Join the webinar and Q&A live on September 15th or watch the replay.